Introduction
The integration of artificial intelligence (AI) in healthcare has led to significant advancements, and one of the most impactful technologies is Natural Language Processing (NLP). NLP, a subset of AI, focuses on the interaction between computers and human language. In clinical trials, NLP is proving to be a game-changer, enhancing various aspects from data extraction to patient recruitment. This article explores the critical role of NLP in clinical trials, detailing its benefits, applications, and the challenges it faces.
Understanding Natural Language Processing (NLP)
What is NLP?
Natural Language Processing (NLP) involves the use of algorithms and computational methods to analyze and interpret human language. It allows machines to understand, interpret, and generate human language in a way that is both meaningful and useful.
Key Components of NLP:
- Tokenization: Breaking down text into smaller units, such as words or phrases.
- Sentiment Analysis: Determining the emotional tone behind a series of words.
- Named Entity Recognition (NER): Identifying and classifying key elements in text into predefined categories such as names of people, organizations, locations, and medical terms.
- Part-of-Speech Tagging: Identifying parts of speech for each word in a sentence.
- Syntax and Semantic Analysis: Understanding the structure and meaning of sentences.
NLP in Clinical Trials
Enhancing Data Extraction and Management
Clinical trials generate vast amounts of unstructured data from various sources, including patient records, clinical notes, and research papers. NLP can efficiently extract relevant information from these texts, transforming unstructured data into structured, usable formats.
- Automated Data Extraction: NLP algorithms can sift through large datasets, extracting critical information such as patient demographics, medical histories, and trial outcomes.
- Improved Data Quality: By standardizing data extraction processes, NLP reduces human error, ensuring higher accuracy and reliability of data.
Facilitating Patient Recruitment
Finding suitable participants for clinical trials is often a challenging and time-consuming process. NLP can streamline patient recruitment by analyzing medical records and identifying potential candidates who meet the trial’s inclusion criteria.
- Precision Matching: NLP tools can match patient profiles with trial requirements more accurately than manual methods.
- Enhanced Outreach: NLP can help design targeted communication strategies to reach potential participants, increasing engagement and reducing recruitment time.
Improving Patient Monitoring and Adverse Event Reporting
Continuous monitoring of trial participants is crucial for assessing treatment efficacy and safety. NLP can aid in real-time monitoring and reporting of adverse events.
- Real-Time Analysis: NLP systems can analyze patient feedback and clinical notes in real-time, identifying potential adverse events early.
- Automated Reporting: NLP can automate the reporting process, ensuring timely and accurate documentation of adverse events.
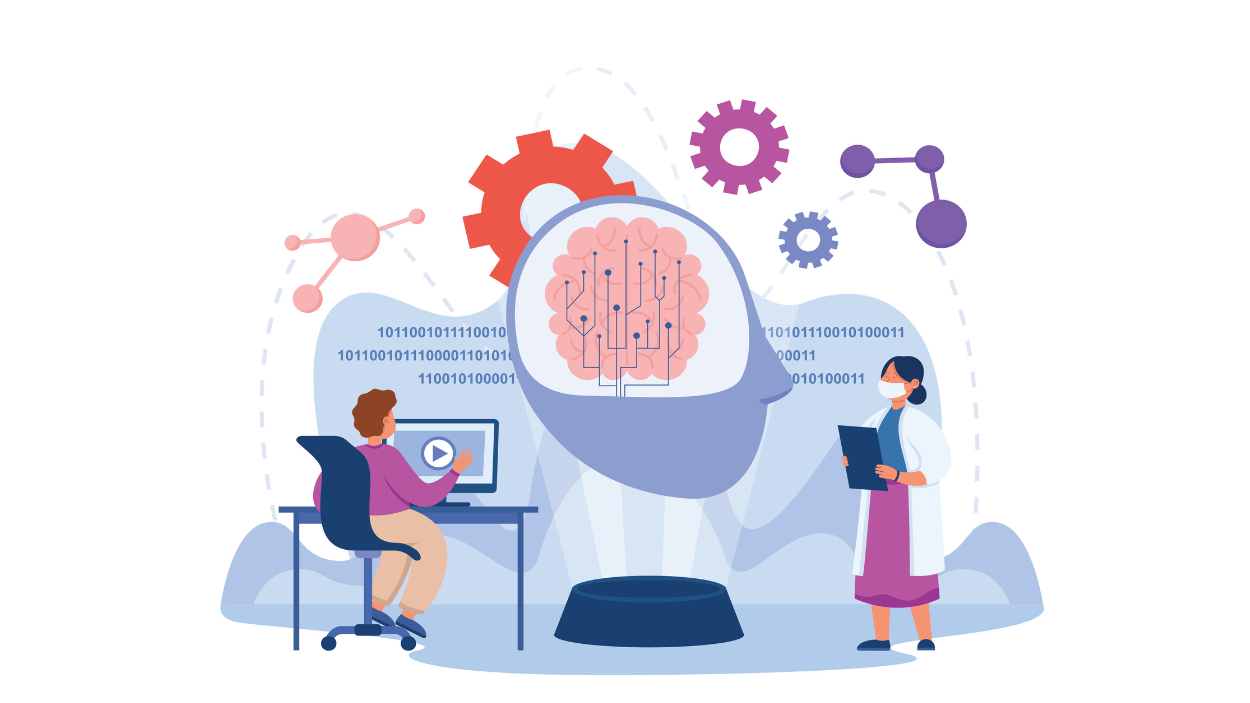
Applications of NLP in Clinical Trials
Literature Review and Synthesis
NLP can automate the process of reviewing and synthesizing scientific literature, saving researchers significant time and effort.
- Automated Literature Search: NLP tools can scan thousands of research papers, identifying relevant studies and summarizing key findings.
- Evidence Synthesis: NLP can help synthesize evidence from multiple studies, providing comprehensive insights and identifying research gaps.
Protocol Development and Compliance
Developing and adhering to clinical trial protocols is essential for regulatory compliance and trial success. NLP can assist in protocol development and monitoring.
- Protocol Optimization: NLP can analyze existing protocols and suggest improvements based on historical data and current research.
- Compliance Monitoring: NLP tools can monitor trial activities and documentation for protocol adherence, flagging potential compliance issues.
Enhancing Data Interpretation and Insights
NLP can transform raw data into meaningful insights, supporting decision-making processes in clinical trials.
- Trend Analysis: NLP in conjunction with other algorithms, including those from the fields of machine learning and deep learning, can identify trends and patterns in clinical data, helping researchers understand treatment effects and patient responses.
- Predictive Analytics: NLP can support predictive modeling, forecasting outcomes based on historical data and current findings.
Challenges of Implementing NLP in Clinical Trials
Data Privacy and Security
Handling sensitive patient data requires strict adherence to privacy regulations and robust security measures.
- Regulatory Compliance: Ensuring compliance with data protection regulations such as DPDPA is crucial.
- Data Anonymization: NLP systems must anonymize patient data to protect privacy while maintaining data utility.
Handling Unstructured Data
Clinical data is often unstructured and varies in quality, posing challenges for NLP systems.
- Data Standardization: Standardizing data formats and terminology is essential for effective NLP implementation.
- Noise Reduction: NLP tools must be capable of filtering out irrelevant information and focusing on meaningful data.
Algorithm Bias and Accuracy
NLP algorithms are only as good as the data they are trained on, and biases in training data can lead to skewed results.
- Bias Mitigation: Continuous monitoring and updating of NLP models are necessary to minimize bias.
- Accuracy Improvement: Ensuring high accuracy in data extraction and interpretation is critical for reliable outcomes.
Future Prospects of NLP in Clinical Trials
The future of NLP in clinical trials looks promising, with advancements in AI and machine learning paving the way for more sophisticated applications.
- Integration with AI: Combining NLP with other AI technologies can enhance its capabilities, enabling more comprehensive data analysis and insights.
- Personalized Medicine: NLP can support the development of personalized treatment plans by analyzing individual patient data and predicting responses to therapies.
- Global Collaboration: NLP can facilitate global collaboration in clinical research, allowing researchers to share and analyze data across borders.
Conclusion
Natural Language Processing (NLP) is revolutionizing the landscape of clinical trials by enhancing data extraction, patient recruitment, and monitoring processes. Despite challenges such as data privacy, handling unstructured data, and algorithm bias, the benefits of NLP are undeniable. As technology continues to advance, the role of NLP in clinical trials is set to expand, driving innovations and improving patient outcomes.